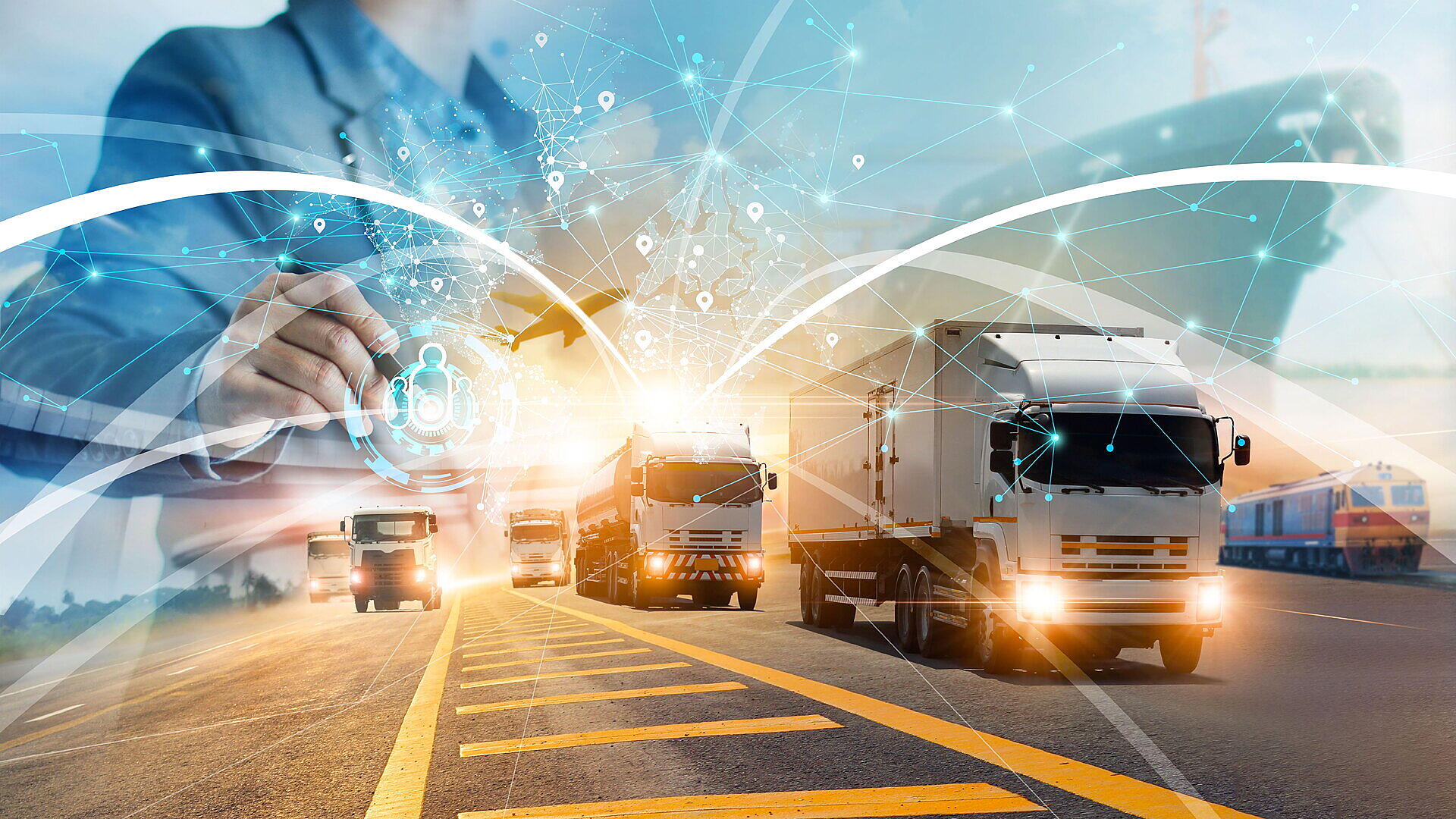
DATA SCIENCE IN FREIGHT TRANSPORTATION
Data science is the use of tools and techniques to study numerical data, identify hidden patterns, extract valuable insights, and make informed business decisions. This field leverages various technologies, including analytical software (e.g. Python), the Internet of Things (IoT), artificial intelligence (AI), and machine learning (ML) algorithms, to develop predictive models.
The extent of the application of data science remains unexplored. Data science is becoming increasingly influential in freight transportation, as it can help businesses optimise their supply chain operations, improve efficiency, reduce costs, and stay competitive.
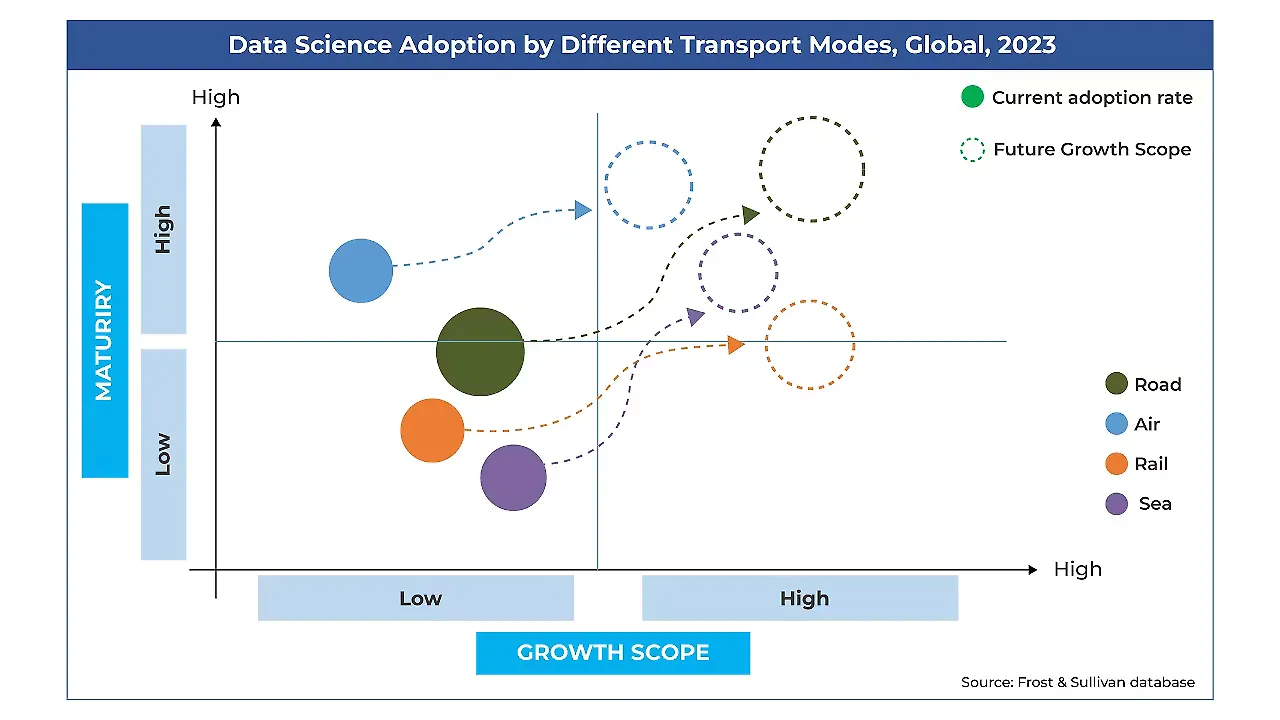
Global MNCs are early adopters of data science technologies. Initial adopters like a package delivery and express mail service company launched a smart trucking solution equipped with IoT sensors to provide real-time data analysis for route optimisation and claims to reduce transit times by up to 50% and help in increasing efficiency and saving fuel consumption.
An integrated container logistics provider (Maersk) started offering a centralised dashboard solution, an AI-powered mapped tool that provides details of ports, live location of the vessel, journey map, pricing map of different carriers, import and export overview, expected time of arrival (ETA), and live updates.
Domestic logistic providers, especially start-up companies, are trying to catch up with data science technology. A new-age relay transport company uses data science to solve truck driver shortage problems and help improve lead time and increase truck efficiency.
A company focusing on e-commerce logistics has developed a platform powered by AI, which gathers large numbers of data involving addresses, products, customer behaviour, vehicular movement, utilisation, and operations of facilities for better service quality and success delivery rate.
It also gives importance to its network design for competitiveness. It has developed an AI and ML-driven network optimisation engine that analyses historical data along with current market trends to predict the performance of its multiple networks’ configuration to upgrade and maintain the most optimal network design in a shorter period.
A start-up company offering 10-minute grocery delivery services analyses the buying pattern of the consumers, purchasing power, and total spending power to develop business strategies. For route optimisation, it analyses traffic routes to get the average cycle time for delivery in a particular area.
Frost & Sullivan sees increasing data science spending by domestic companies to stay competitive and help its customers with better service.
DATA SCIENCE AIDING IN SOLVING MAJOR CHALLENGES IN TRANSPORTATION
Companies have adopted data science to gain a competitive edge, optimise their processes, cut expenses, and offer improved customer services. Some of the applications of data science are:
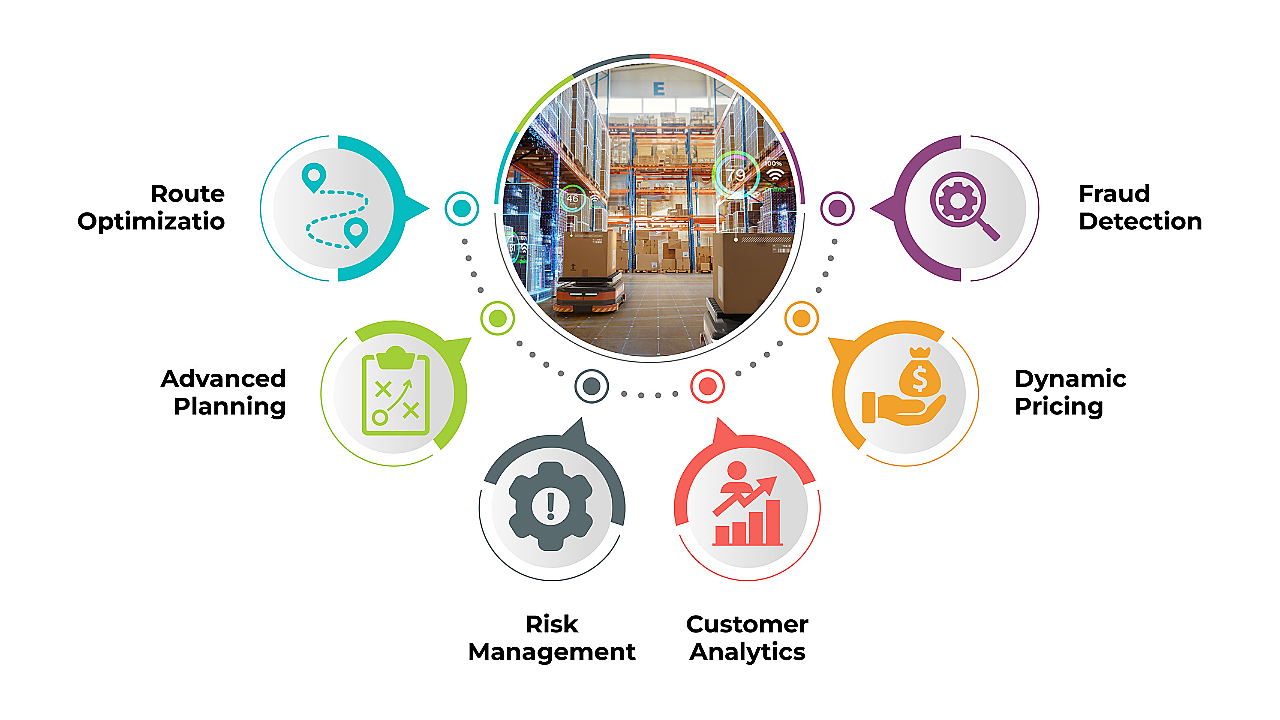
- Route optimisation: Data science can help logistics companies optimise delivery routes by analysing traffic patterns, historical data, weather conditions, and other factors to find the most efficient routes for drivers. This can save time and money, reduce fuel consumption, and improve delivery times.
- Advanced planning: Large inventory, slow and non-moving goods play a major role in improving the cash flow situation of a company. Companies are using data science to find hidden patterns that help in better forecasting and improving cash flow in general.
- Risk management: Logistics businesses can spot possible issues before they arise and take preventative action by analysing data from numerous sources, including sensors and global positioning system (GPS) devices.
- Customer analytics: Data science enables logistics companies to examine data on buying trends, tastes & behaviour, and assists them in better understanding customers. This can help them tailor their services to meet customers’ needs more effectively.
- Dynamic pricing: Using real-time data such as traffic, distance, fuel prices, market demand, competition, and weather forecasts, freight carriers can adjust their pricing strategy. This helps them maximise their revenue while still offering competitive rates to customers and optimise their capacity utilisation.
- Fraud detection: By leveraging data analytics and other technologies, companies can detect and prevent fraudulent activities such as cargo theft or fraudulent billing. This can help freight transportation companies reduce financial losses, improve customer trust, and enhance security measures. For instance, in certain cases, a company might charge more than other companies for the same route. In this situation, businesses can find the hidden historical pattern, analyse the price shift trend, and adapt their cost accordingly.
FUTURE APPLICATIONS OF DATA SCIENCE IN TRANSPORTATION
Data science application is bound to increase in the future, and more applications/ areas are getting developed and researched. A few areas where data science will be adopted in the next few years include:
- Driver safety: Data science can be used to prevent accidents or loss by tracking driver behaviour, such as exceeding the speed limit or harsh driving practices and alerting the driver or managers to take immediate action.
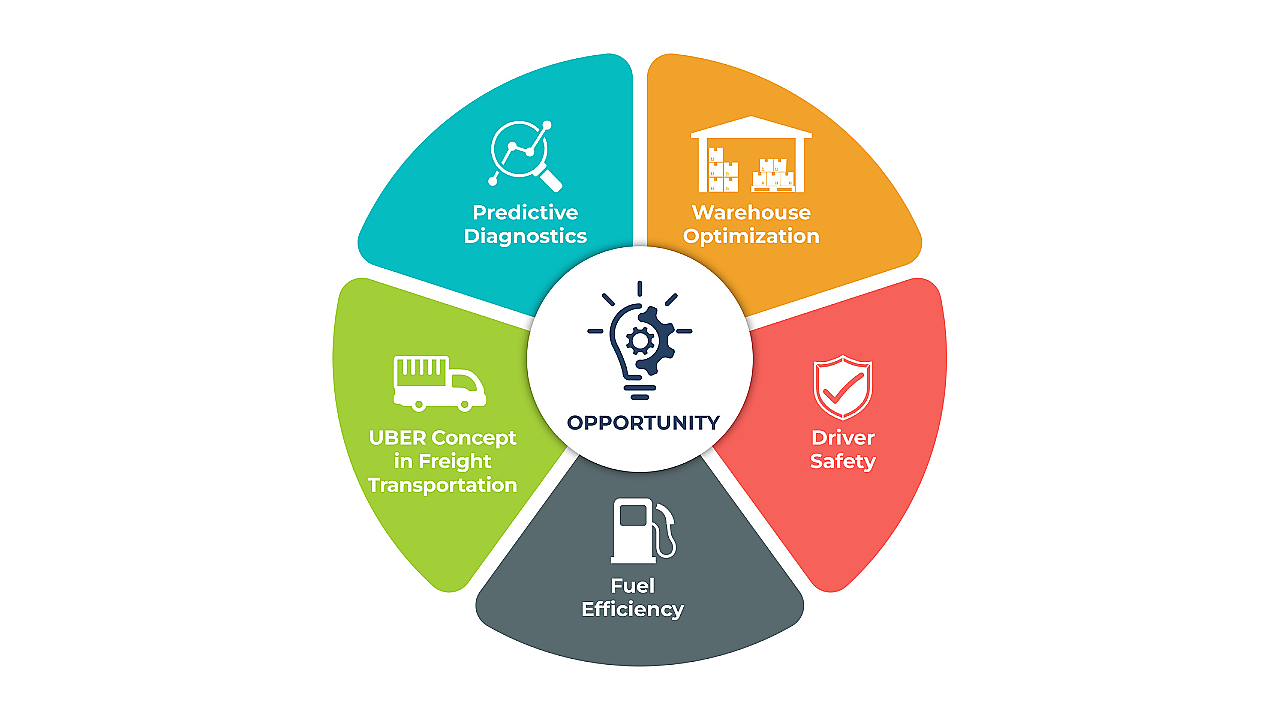
- Fuel efficiency: Data science can optimise fuel consumption by tracking if the driver takes unnecessary route deviation or has bad driving practices. Usually, a vehicle performs best in certain weather conditions, speed, and braking patterns. Freight companies can educate drivers on these lines to gain additional fuel efficiency.
- UBER concept in freight transportation/foresight: UBER is a pioneer in using data science to predict future demand for taxi drivers to maximise their vehicle utilisation. Moving onward, we see fleet transportation adopt these techniques to better utilise their assets.
- Warehouse optimisation: A warehouse is a capex intensive part of logistics. In places like India, space is a costly commodity. Understanding hidden patterns in customer demand will help these infrastructure companies to implement advanced shared services solutions, which will reduce customer costs and improve asset utilisation.
- Predictive diagnostics (prognosis): In the supply chain journey, the most important asset of a company is the vehicle. Transport companies do not like any breakdown of vehicles. In this situation, data science can analyse vehicle data, such as engine performance and tyre wear, to predict when a part of the vehicle is likely to experience failure and under what conditions. Predictive diagnostics will help the company pre-empt action to avoid vehicle idle time.
INDIA AS A DATA SERVICE HUB FOR FREIGHT TRANSPORTATION
For any freight company, extracting valuable information from data is a long and expensive process requiring significant human resource investments. India has been a source for global companies to provide quality Information Technology Enabled Services (ITES) by providing trained manpower at competitive rates.
The Indian Government is very proactive and focused on modernising transport infrastructure in the country, and technology spending is gaining momentum. We see an opportunity for Indian ITES service providers to use this opportunity to fill the resource gap and provide data science services to all transportation companies across the globe.
The opportunity can bring standardisation in technology, help small-scale logistics companies adopt it, and help them be competitive in the larger environment. India’s freight transportation software sector is expected to continue growing in the years ahead as businesses seek to optimise their supply chains and improve their competitiveness.
About the Author: Soumya Sinha is Director, Supply Chain and Logistics Practice, MEASA at Frost & Sullivan
Also Read
Data Is The New Oil For Automakers - Nesh LIVE’s Venkat Nathan